- Accueil >
- Publications >
- An [18F]FDG-PET/CT deep learning method for fully automated detection of pathological mediastinal lymph nodes in lung cancer patients
An [18F]FDG-PET/CT deep learning method for fully automated detection of pathological mediastinal lymph nodes in lung cancer patients
Auteurs
David Wallis, Michaël Soussan, Maxime Lacroix, Pia Akl, Clément Duboucher, Irène Buvat
Résumé
Abstract
Purpose
The identification of pathological mediastinal lymph nodes is an important step in the staging of lung cancer, with the presence of metastases significantly affecting survival rates. Nodes are currently identified by a physician, but this process is time-consuming and prone to errors. In this paper, we investigate the use of artificial intelligence–based methods to increase the accuracy and consistency of this process.
Methods
Whole-body
Results
On the test set from the first scanner, our model achieved a sensitivity of 0.87 (95% confidence intervals [0.74, 0.94]) with 0.41 [0.22, 0.71] false positives/patient. This was comparable to the performance of an expert. Without transfer learning, on the test set from the second scanner, the corresponding results were 0.53 [0.35, 0.70] and 0.24 [0.10, 0.49], respectively. With transfer learning, these metrics were 0.88 [0.73, 0.97] and 0.69 [0.43, 1.04], respectively.
Conclusion
Model performance was comparable to that of an expert on data from the same scanner. With transfer learning, the model can be applied to data from a different scanner. To our knowledge it is the first study of its kind to go directly from whole-body [18F]FDG-PET/CT scans to pathological mediastinal lymph node localisation.
Membres
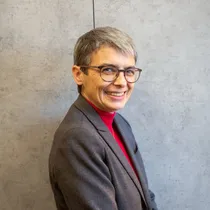