- Accueil >
- Publications >
- MnM: a machine learning approach to detect replication states and genomic subpopulations for single-cell DNA replication timing disentanglement
MnM: a machine learning approach to detect replication states and genomic subpopulations for single-cell DNA replication timing disentanglement
Auteurs
Joseph M. Josephides, Chun-Long Chen
Résumé
ABSTRACT
We introduce MnM, an efficient tool for characterising single-cell DNA replication states and revealing genomic subpopulations in heterogeneous samples, notably cancers. MnM uses single-cell copy-number data to accurately perform missing-value imputation, classify cell replication states and detect genomic heterogeneity, which allows to separate somatic copy-number alterations from copy-number variations due to DNA replication. By applying our machine learning methods, our research unveils critical insights into chromosomal aberrations and showcases ubiquitous aneuploidy in tumorigenesis. MnM democratises single-cell subpopulation detection which, in hand, enables the extraction of single-cell DNA replication timing (scRT) profiles from genomically-heterogenous subpopulations detected by DNA content and issued from single samples. By analysing over 119,000 human single cells from cultured cell lines, patient tumours as well as patient-derived xenograft samples, the copy-number and replication timing profiles issued in this study lead to the first multi-sample subpopulation-disentangled scRT atlas and act as data contribution for further cancer research. Our results highlight the necessity of studying
GRAPHICAL ABSTRACT
Equipes
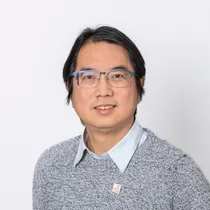
Membres
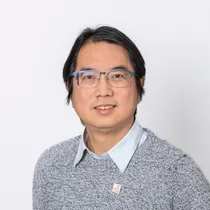