- Accueil >
- Recherche et innovation >
- Nos équipes >
- Equipes et groupes de recherche
Les équipes et groupes de recherche
Le Centre de recherche de l’Institut Curie rassemble 86 équipes de recherche et des groupes de recherche translationnelle. Leurs travaux multidisciplinaires et interdisciplinaires s’inscrivent dans les domaines de la biologie, chimie, physique, chemical biology, physique des systèmes vivants, biologie cellulaire, biologie computationnelle, biologie du développement, biologie des tumeurs, radiobiologie, génétique, épigénétique, immunologie et imagerie moléculaire.
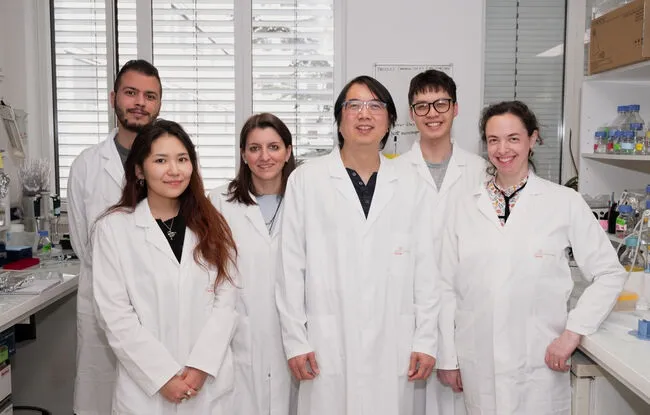
Trouver une équipe de recherche
Filtrer
Filtrer
Axes de recherche thématiques
Unités
Responsables
Labels
Trouver une équipe de recherche
93 résultat(s)